역전파는 신경망을 훈련하기 위해 딥 러닝에 사용되는 기본 알고리즘입니다. 네트워크 예측의 오류를 줄이기 위해 네트워크의 가중치를 업데이트하는 데 사용됩니다. 이 페이지에서는 역전파와, 곱셈 계층, 덧셈 계층 역전파의 특징과 구현에 대해 설명합니다.
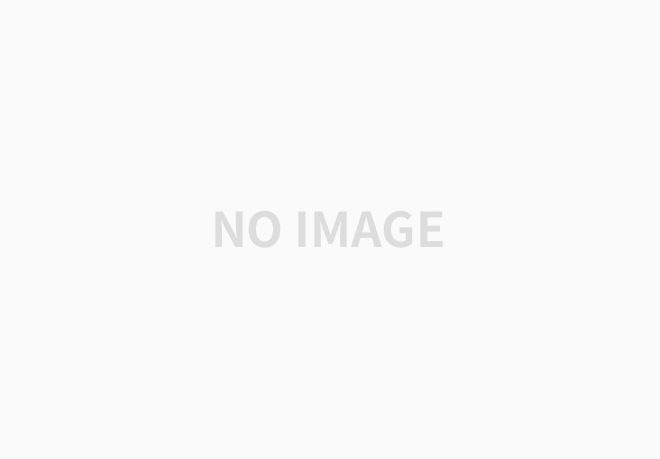
역전파 특징
역전파의 기본 아이디어는 네트워크의 각 가중치에 대한 오차의 기울기를 계산하는 것입니다. 그래디언트는 각 가중치를 변경할 때 오류가 얼마나 변경되는지 알려주고 오류를 줄이는 방식으로 가중치를 업데이트할 수 있게 해줍니다.
역전파 알고리즘은 네트워크의 현재 가중치로 예측하는 것으로 시작합니다. 그런 다음 예측을 실제 출력과 비교하고 오류를 계산합니다. 그런 다음 오류는 입력 계층에 도달할 때까지 계층별로 네트워크를 통해 역방향으로 전파됩니다.
각 계층에서 오류는 해당 계층의 각 가중치에 대한 오류의 기울기를 계산하는 데 사용됩니다. 이러한 그래디언트는 오류를 줄이는 방식으로 가중치를 업데이트하는 데 사용됩니다. 이 프로세스는 네트워크 예측 오류가 충분히 낮아질 때까지 여러 번 반복됩니다.
역전파의 주요 이점 중 하나는 많은 계층이 있는 심층 신경망을 훈련할 수 있다는 것입니다. 이는 오류가 네트워크를 통해 계층별로 전파되어 각 계층이 오류에 따라 가중치를 업데이트할 수 있기 때문입니다.
역전파는 강력한 알고리즘이며 이미지 분류, 자연어 처리 및 음성 인식과 같은 다양한 작업을 수행하도록 심층 신경망을 훈련시키는 데 널리 사용되었습니다.
요약하면 역전파는 신경망의 각 가중치에 대한 오차의 기울기를 계산하고 오차를 줄이는 방식으로 가중치를 업데이트하여 딥러닝 신경망을 훈련시키는 데 사용되는 알고리즘입니다. 딥 러닝에 널리 사용되며 다양한 작업에서 최첨단 결과를 달성하는 데 사용되었습니다.
역전파 구현
import numpy as np
# Define the sigmoid function for activation
def sigmoid(x):
return 1 / (1 + np.exp(-x))
# Define the derivative of the sigmoid function
def sigmoid_derivative(x):
return x * (1 - x)
# Define the input and output data
X = np.array([[0, 0], [0, 1], [1, 0], [1, 1]])
y = np.array([[0], [1], [1], [0]])
# Define the number of iterations and the learning rate
num_iterations = 20000
learning_rate = 0.1
# Define the number of input and output nodes
input_nodes = 2
output_nodes = 1
# Define the weights for the input-hidden and hidden-output layers
weights_ih = np.random.rand(input_nodes, 2)
weights_ho = np.random.rand(2, output_nodes)
# Train the network
for i in range(num_iterations):
# Forward propagate the input through the network
input_layer = X
hidden_layer = sigmoid(np.dot(input_layer, weights_ih))
output_layer = sigmoid(np.dot(hidden_layer, weights_ho))
# Calculate the error for the output layer
output_error = y - output_layer
output_delta = output_error * sigmoid_derivative(output_layer)
# Calculate the error for the hidden layer
hidden_error = np.dot(output_delta, weights_ho.T)
hidden_delta = hidden_error * sigmoid_derivative(hidden_layer)
# Update the weights for the input-hidden and hidden-output layers
weights_ho += np.dot(hidden_layer.T, output_delta) * learning_rate
weights_ih += np.dot(input_layer.T, hidden_delta) * learning_rate
# Print the final output of the network
print(output_layer)
이 코드는 활성화를 위해 시그모이드 함수를 사용하는 숨겨진 레이어가 하나 있는 간단한 신경망을 정의합니다. 입력 데이터 X 및 y, 반복 횟수 및 학습률은 코드 시작 부분에 정의됩니다.
순방향 전파는 첫 번째 섹션에서 수행되며 오류는 예상 값에서 출력을 빼서 계산됩니다. 그런 다음 오류는 체인 규칙을 사용하여 역전파되어 숨겨진 계층에 대한 오류를 계산하고 그에 따라 가중치를 업데이트합니다.
이것은 역전파의 간단한 예이며 특정 문제, 네트워크의 크기와 계층 수, 활성화 기능에 맞게 코드를 조정해야 한다는 점에 유의해야 합니다.
'컴퓨터과학/딥러닝' 카테고리의 글 목록
모든 분야의 정보를 담고 있는 정보의 호텔입니다. 주로 컴전기입니다.
jkcb.tistory.com